By Lisa M.P. Munoz
If you had asked me to draw an engineer when I was in middle school, I would have drawn my big sister. I would go on to pursue engineering just like her. She chose that path after watching informational college videos, designed to recruit women into the profession, showing how much engineers could make a difference in society.
That’s the power of pictures. They shape how we think the world works, who we admire, and, although sometimes unconsciously, who we think we’ll become one day. As society evolves, so too do the pictures we create, whether in film or on paper. For decades, social scientists have documented those changes in perceptions of scientists and engineers in a series of experiments — increasingly reflecting broader views of STEM than previous generations.
These experiments have shown major shifts in children’s perceptions of scientists over the last 60 years. But recent breakthroughs in artificial intelligence (AI) and increased use of AI tools are raising questions about what images future generations will see and how that may impact future generations of scientists. Rather than freeing us from past stereotypes, new research suggests these high-tech images are yet another data set that mirrors society’s deeply entrenched human biases.
Draw a Scientist, analog version
Started in the 1960s, the Draw a Scientist studies were relatively simple — ask children at different ages to draw a scientist and then code for different features of the drawings such as gender. Researchers had to rely on features like hair length and attire, as well as sometimes ask children about the drawing, to help determine the intended gender.
The initial studies showed that children overwhelmingly viewed scientists as male. In the first 10 years of the study, less than 1% of kids drew a female scientist.
In 5,000 drawings collected over the first 11 years of the study, only 28 of them depicted a female scientist, and all of these were drawn by girls. Not a single boy in the study drew a female scientist. Most scientists were depicted wearing lab coats.
Fast forward to 2018 and a large meta analysis of Draw a Scientist studies showed that the share of drawings appearing to depict females had grown to about 33%, a major shift from the 1% in the 1960s. They still found that one in two drawings showed a scientist in a lab coat, and 8 in 10 depicted the scientist as white.
Interestingly, the researchers have found that as students aged from kindergarten through high school, they drew fewer female scientists, perhaps reflecting the societal inputs that signaled that STEM was not a traditional path for women.
My fifth-grade daughter’s science teacher has been doing Draw a Scientist exercises since 1995 and has also seen a shift — more children drawing pictures of scientists with their own gender and ethnicity, as well as more boys drawing women scientists, fewer lab coats, and more scientists outside.
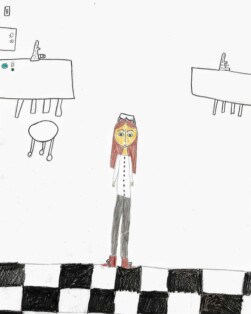
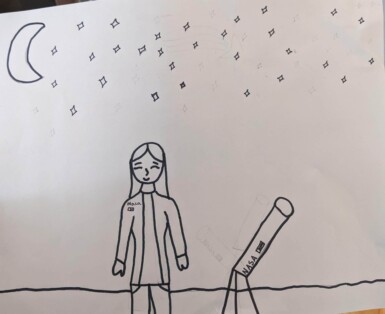
These changes represent real shifts in how individuals, and likely society at large, view not only who can be a scientist, but also how science works. It’s a very slow shift but one nonetheless — away from the lone white, male scientist working in a lab to the view that anyone can do science anywhere.
So, now that we have a baseline of how humans picture a scientist and how that has changed over time, let’s explore how computers picture a scientist.
Draw a Scientist, AI version
To be clear, I know that computers, even advanced AI, do not dream or imagine things independent of their human programming and training datasets. Modern AI tools reflect the input that humans give them, and with ChatGPT and other large language models (LLMs), these powerful programs now have vast amounts of data at their disposal.
At a recent meeting of the National Association of Science Writers, I learned about a fascinating recent study in the Lancet in which researchers asked a large language model to invert stereotypical images from public health to create new AI-generated images.
They prompted the program with things like “African doctors administer vaccines to poor white children in the style of photojournalism” and “Black African doctor is helping poor and sick white children, photojournalism,” and it did not compute. The program would not match the requested race in all but one image. While it would generate images of a group of Black doctors or a group of white children in need, it appeared unable to pair them together.
Inspired by this fascinating and disturbing experiment, I undertook one of my own. I asked the AI-image generation model Stable Diffusion to draw 100 images of a ”photorealistic scientist.” Below is a composite of some of those images. Shockingly, only 6 of 100 depicted what appeared to be a woman scientist. Most appeared older and white. (Note: this was nonscientific coding, based on my casual analysis.) The model’s pictures were closer to the children of the 1960s than those of 2018.

What about engineering?
In 2004, researchers created a Draw an Engineer test, modeled after the Draw a Scientist studies to examine students’ perceptions of what engineers do. At the time, the prevailing stereotype of an engineer was as a train operator.
In one study, the researchers asked for both drawings and written descriptions from 384 Massachusetts children in grades 3 through 12 and, like in Draw a Scientist, looked at features like gender. They also studied features like tools, cars, computers, desks, and trains, finding that most students’ description thematically fit into “building and fixing,” followed by “design.”
Many of the drawings were of stick figures, but of the 64 drawings with evidence of gender, 61% signaled stereotypically male-associated features (e.g. short hair, necktie), while 39% signaled stereotypically female-associated features (e.g. long hair).
As with the Draw a Scientist, female students were more likely to draw females than males. Interestingly, most drawings that featured women came from a classroom in which two female undergraduate engineering students had been working with the younger students.
A later study in 2018 of 75 students in Turkey found that 56% of students drew engineers who appeared male, while about 17% drew female engineers (the others drew symbols of engineering rather than people). Not a single male student drew a female engineer.
So, what about AI? What images does it generate when asked to draw an engineer?
Interestingly, when prompted with “engineer,” the AI image generator not only created images of human engineers but, like the Turkish students, also generated symbols of engineering, like circuit diagrams or blueprints. To filter for this, I asked it to generate “photorealistic” images (which is why I did so for the scientists, too, to have parallel datasets).
Of the 100 images showing engineers, 1 appeared to be female. Like for the AI-generated scientists, most appeared older and male. It was again like being back in the 1960s.

Where do we go from here?
My casual AI experiment revealed that the vast majority of images of scientists and engineers that the LLM are being trained on are majority white male. Combined with the Lancet study on public health images, this data exposes the deep and persistent human biases that still plague public perceptions of STEM fields.
While humans have made great strides in their pictures of scientists, AI is still decades behind. Our best defense is to keep elevating the stories of successful women scientists and engineers from the last several decades. Telling the stories of these women in STEM can help shape not only human perceptions of the fields, but also — eventually — AI ones. Most importantly, these stories can help inspire and empower the next generation of innovators, working to make a difference in the world.
Author
-
Lisa M.P. Munoz is a science writer, SWE member, and author of “Women in Science Now: Stories and Strategies for Achieving Equity” (Columbia University Press, October 2023). President of SciComm Services, a science communications consulting firm, Munoz is a former journalist and press officer with more than 20 years of experience crafting science content for scientists and the public alike. Munoz holds an engineering degree from Cornell University.